In the field of modern recruitment, the use of artificial intelligence and algorithms is rapidly popularising. From screening resumes to evaluating interview performance, algorithms seem to be able to select the best candidates efficiently and impartially.However,algorithmic systems can yield socially-biased outcomes, thereby compounding inequalities in the workplace and in society(Kordzadeh, N. and Ghasemaghaei, M.,2021).
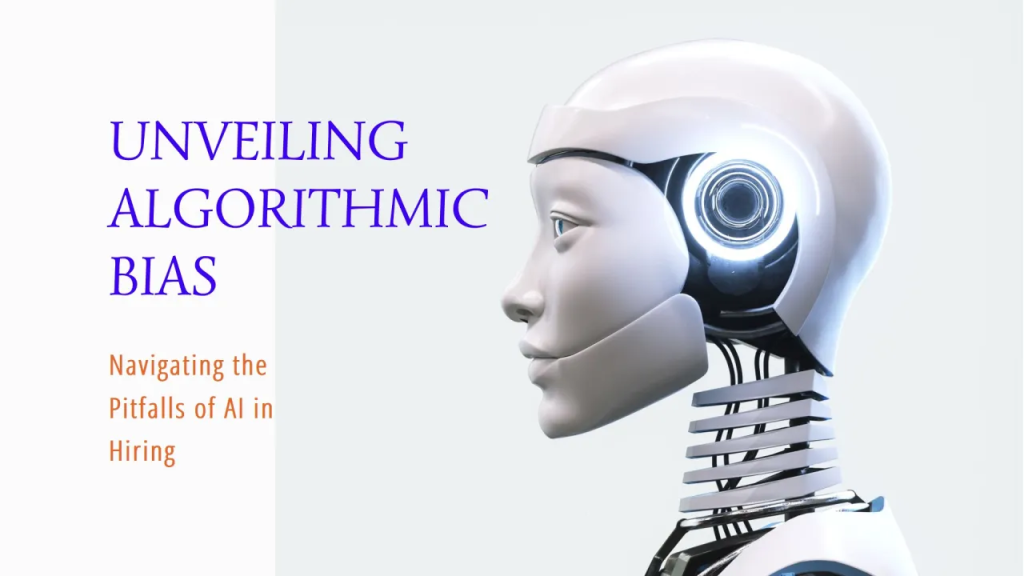
Source of algorithm bias in recruitment
With the popularisation of artificial intelligence technology, algorithms are widely used in the recruitment process, which is used to screen resumes, match positions and evaluate candidates. Although the original intention of these tools is to improve efficiency and reduce human bias, in reality, algorithmic bias is not only widespread, but may also exacerbate existing inequality. Algorithm bias in recruitment is not a simple technical problem, but a combination of multiple factors such as data, design and social culture.
- The bias of historical data
Algorithms rely on historical data for training, which often reflect the discrimination and injustice in past recruitment decisions. For example, in some industries, the proportion of men in technical positions is significantly higher than that of women, which makes the algorithm tend to regard “men” as a key feature of technical positions. Similarly, some ethnic, regional or college backgrounds may be misjudged by the algorithm as “non-standard” due to the low recruitment rate. This bias has led to recruitment tools inadvertently strengthening the historical discrimination model and continuing inequality to modern times.
Amazon’s recruitment system uses the company’s employment data within ten years for training, resulting in the algorithm to automatically deduct points for resumes containing “female”-related words (such as “women’s college” and “women’s society”), which is indirectly gender discrimination.
- 2.Insufficient data representativeness
If the training data of the algorithm lacks full representation of candidates from women, ethnic minorities or non-traditional backgrounds, these groups will be considered abnormal or inappropriate. For example, many enterprises rely too much on data samples of graduates of famous schools when training recruitment algorithms, resulting in algorithms to underestimate the ability of excellent candidates from other colleges and universities. This lack of representation further marginalizes vulnerable job seekers, further reducing the fairness of recruitment.
- 3.Implicit bias in feature selection
Which variables need to be selected as the basis for decision-making when designing algorithms, and the selection process of these variables often hides the subjective assumptions of the designer. For example, the algorithm may take “stability” as a key feature and prioritise candidates with continuous career experience, which will have an adverse impact on women who interrupt their careers due to childcare and other reasons. In addition, gender stereotypes may also penetrate into the selection of characteristics, such as paying more attention to “competitiveness” in men’s resumes and ignoring “teamwork” in women’s resumes and other characteristics. This bias is not only a technical problem, but also reflects a deep cultural bias.
Consequences of recruitment algorithm bias: the hidden impact of exacerbating inequality in the workplace
In the field of modern recruitment, algorithms are rapidly replacing the traditional human resources process. From resume screening to candidate evaluation, artificial intelligence technology is considered efficient and “objective”. However, this is not the case.Algorithmic biases can lead to significant harms and injustices, particularly if less-important moral values are prioritized (Fazelpour, S. and Danks, D.,2021).It may unconsciously exacerbate inequality in the workplace, resulting in the deprivation of employment opportunities for certain groups, and have a profound impact on individuals, enterprises and even society.
- Deprivation of job opportunities: the expansion of implicit discrimination
The main source of algorithm bias is training data. If the data contains historical biases on gender, race or age, the algorithm will “learn” and continue it. For example, some recruitment systems tend to reduce the score of women’s career interruptions due to parenting, or infer the racial background of candidates based on their names and areas of residence, thus affecting recruitment decisions. This invisible bias makes some groups systematically marginalised in the job search process and unable to obtain equal opportunities for competition.
- Innovation is hindered
Diversity is an important factor in driving enterprise innovation. Research shows that diverse teams can come up with innovative ideas and make effective decisions. However, bias algorithms tend to prefer candidates with traditional backgrounds, such as men, graduates of specific colleges or certain racial groups. This not only causes enterprises to miss employees with diverse perspectives, but also may weaken their competitiveness in the global market. McKinsey’s research points out that the performance of teams with high diversity is often higher than the industry average, and the bias algorithm will undoubtedly make enterprises “discard their martial arts skills” at this point.
- Strengthen the stereotype in the workplace culture
When the algorithm prioritises the admission of specific types of candidates, the workplace culture will also become more single. For example, male-dominated technical teams will further exclude women due to algorithmic screening, thus forming a xenophobic working environment. This phenomenon not only makes it difficult for the team to accept different views, but also leads to the further strengthening of gender and racial stereotypes, making it more difficult to build diversity in the workplace.
- Damage the reputation and legal risks of the enterprise
With society’s attention to fairness and diversity, algorithmic bias has also become the focus of public opinion and laws and regulations. If an enterprise leads to employment discrimination due to the use of bias algorithms, it will not only face legal proceedings, but also may encounter a public relations crisis, which will seriously damage the brand image.
- Solidify the inequality in the labour market
The widespread use of recruitment algorithms may systematically continue social inequality. Male-dominated industries may further exclude women, while certain races and minorities may be excluded from high-income jobs for a long time. This solidification phenomenon will limit the mobility of society, further widen the income gap between different groups, and make the construction of an equal society more difficult.
The key to solving the problem of algorithm bias in recruitment is to start from multiple levels such as data, algorithm design and corporate culture to ensure fairness and diversity. First of all, enterprises should ensure the diversity of training data and avoid excessive representation of a single group, especially in terms of gender, race, age and other aspects. Through the review and adjustment of historical recruitment data, remove potential biases and introduce samples of candidates from diverse backgrounds. Secondly, when designing algorithms, we should avoid relying too much on stereotypes and traditional characteristics, such as the continuity of educational background or workplace experience, and the selection of features should be optimised according to the actual needs of the position. Enterprises should conduct regular algorithm bias reviews to ensure that the system does not strengthen the discriminatory pattern in history in actual operation. Finally, the recruitment process should pay more attention to the value of diversity, avoid only pursuing efficiency and short-term suitability, and encourage the team to have a broader perspective on the basis of cultural fit. Through these measures, enterprises can not only improve the fairness of recruitment, but also promote innovation and long-term development.
Algorithm bias in recruitment stems from multiple factors of data, design and social culture. These prejudices not only limit the employment opportunities of certain groups, but also may weaken the innovation ability of enterprises and team diversity, while posing a threat to social equity. In order to avoid the further spread of algorithm bias, when designing and using recruitment tools, enterprises must pay attention to the diversity of training data, optimise equity goals, and establish a regular review mechanism to ensure that technology truly serves fairness and efficiency, rather than becoming a booster of inequality.
Reference list
Bloomberg Originals (2022). How AI is Deciding Who Gets Hired. [online] YouTube. Available at: https://youtu.be/6nGM37ThEsU?si=fs5_MflAmeT9bd5x [Accessed 1 Dec. 2024].
Fazelpour, S. and Danks, D. (2021). Algorithmic bias: Senses, sources, solutions. Philosophy Compass, [online] 16(8). doi:https://doi.org/10.1111/phc3.12760.
Kordzadeh, N. and Ghasemaghaei, M. (2021). Algorithmic bias: review, synthesis, and Future Research Directions. European Journal of Information Systems, 31(3), pp.1–22.
Tiktok.com. (2024). TikTok – Make Your Day. [online] Available at: https://vm.tiktok.com/ZGdjp6aw4/ [Accessed 1 Dec. 2024].
Hi! I think your writing does a great job of exploring the problem of algorithmic bias in recruitment. you shows how using AI can lead to unfair hiring practices, especially for women and minorities. The author explains that these biases often come from historical data and the choices made by the people designing the algorithms. The example of Amazon’s hiring system is a strong illustration of how these problems can harm candidates. you also suggests practical solutions, like using diverse training data and regularly checking for bias in algorithms. Overall, I think u did a really great job!