Algorithmic biases refer to systematic and unfair discrimination that occurs when algorithms, especially those used in artificial intelligence (AI), machine learning, and data-driven decision-making, produce outcomes that are prejudiced or biased in ways that disproportionately affect certain groups of people. These biases can emerge from the data the algorithms are trained on, the design choices made during their development, or the way they are implemented and used. Here are some examples in reality that can refer to the concept of Algorithmic biases.
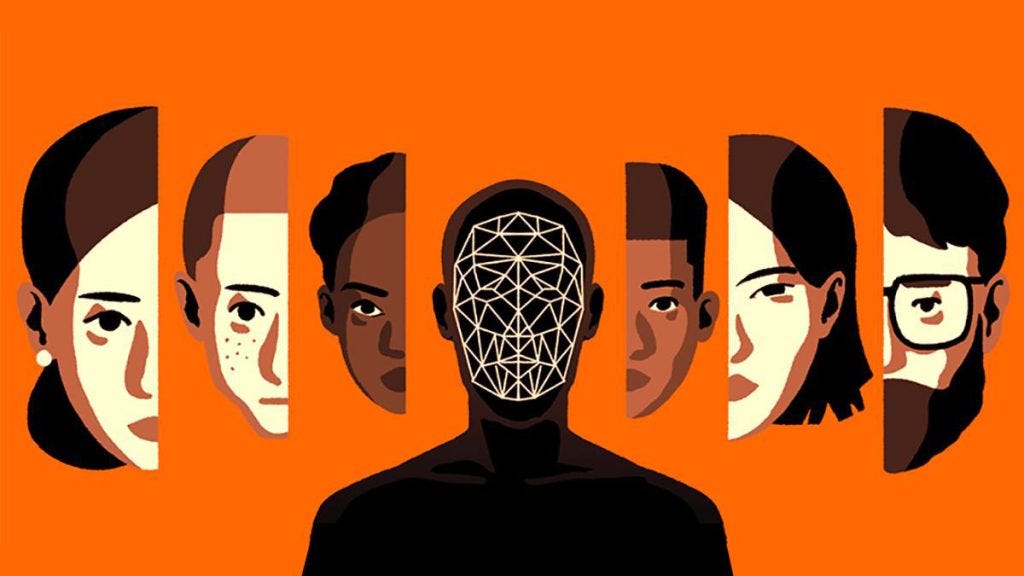
For starters, the facial recognition systems in people’s phones actually have Algorithmic biases. Many facial recognition systems have been shown to perform worse on people with darker skin tones, especially women of color. This is because these systems are often trained primarily on images of lighter-skinned male faces. For example, a 2018 study by the National Institute of Standards and Technology (NIST) found that commercial facial recognition systems misidentified Black and Asian faces at higher rates than white faces. Some systems misidentified dark-skinned women 35% more than light-skinned men. This bias can lead to misidentification, wrongful accusations, and racial profiling, especially in law enforcement contexts, where facial recognition is used for surveillance.
Secondly, Social media platforms use algorithms to recommend content, but these algorithms can be biased based on user engagement, which often leads to type of content. Algorithms on software like Facebook and YouTube can amplify politically biased or extremist content, as it often generates high engagement. This has led to concerns about the spread of misinformation, especially during elections or crises. The bias in algorithmic recommendations could cause lots of different public opinions and spread fake news.
Thirdly, Virtual assistants like Apple’s Siri, Amazon’s Alexa, and Google’s Assistant are often programmed with stereotypical voices or behaviors that improve traditional gender roles. Early versions of Siri and Alexa were programmed to have female voices, often created as subservient or polite, while male virtual assistants are less common. This reinforces the stereotype of women as caregivers or helpers, while men are often associated with authority or expertise. Consequently, The gendered nature of these assistants can contribute to the normalization of traditional gender roles in society, reinforcing unequal between men and women.
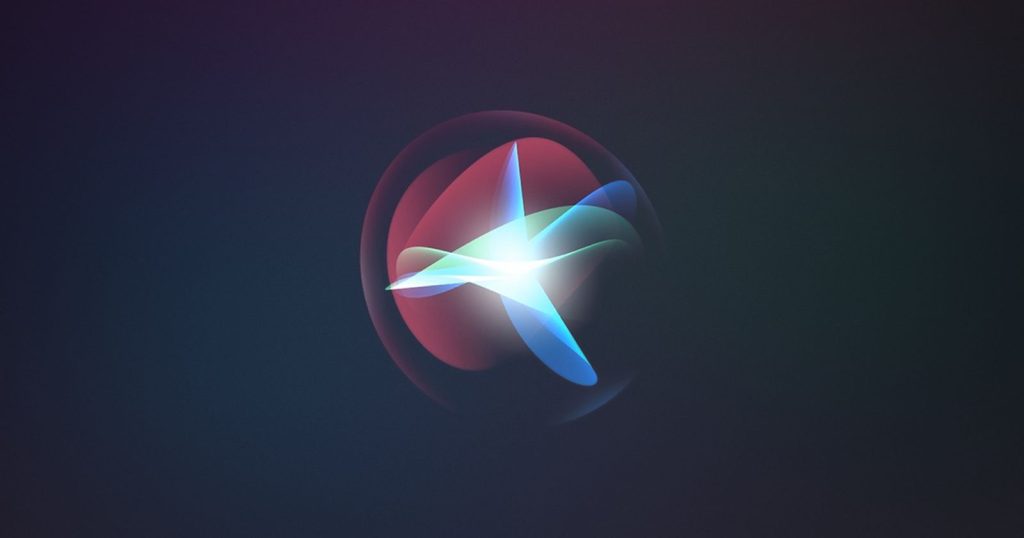
Last but not least, Algorithms used to moderate content on social media platforms can disproportionately affect certain communities, particularly those with non-Western or non-white backgrounds. In 2020, research showed that automated content moderation systems on platforms like Facebook and Instagram often flagged posts from Black activists or marginalized groups as offensive or violating policies more frequently than those from white users. This was due to algorithmic training data that failed to properly account for the nuances of different cultures and languages. This bias can suppress the voices of minority or marginalized groups while allowing harmful content from others to remain unchecked.
In conclusion, algorithmic biases in facial recognition, social media, virtual assistants, and content moderation perpetuate discrimination, reinforcing harmful stereotypes and inequalities, and highlighting the urgent need for fairness and inclusivity in AI development.
This is a great blog, you provide clear and specific examples about the real-life impact of these issues in various fields. You also bring up an important point about the normalisation of stereotypes through technology. Maybe you could list some potential solutions or strategies to mitigate this, such as regular audit systems, that would be helpful.
You did amazing work on this, the examples showing real-life impact, the in-depth explanations of these systems (Especially the facial systems showing racism on social media, something I never even knew about before and I am appalled and disgusted this exists) and it’s very useful for it’s bringing to light the horrible effect that is the normalisation of several harmful stereotypes through technology. It would be nice if you said something we can do about this. Actions against these things, like protests and Boycotts, would be nice for gathering action for the things you’re making people aware of.
The article dissects the impact of algorithmic bias in modern life in a clear and accessible way, not only pointing out the problem, but also proposing some solutions, such as increased transparency and diverse data sets, which gave me concrete direction as I read. In addition, it also provides a way of case analysis, which makes abstract concepts more concrete and easier for me to understand.